Invasion Of The Squares From The Fifth Dimension Mac OS
- Invasion Of The Squares From The Fifth Dimension Mac Os 7
- Invasion Of The Squares From The Fifth Dimension Mac Os Catalina
- Invasion Of The Squares From The Fifth Dimension Mac Os X
The Fifth Dimension Is Through Your Open Heart. Moving into the Fifth Dimension occurs some time after Self Realization. The heart must be open before we can move into the fifth dimension. As is the case with all other spiritual awakening processes, these shifts occur when you are ready, and when you have removed old emotional densities. Search the world's information, including webpages, images, videos and more. Google has many special features to help you find exactly what you're looking for. The 5th Dimension is a dimension in the DC Universe and the home to interdimensional imps, such as Mr. Mxyzptlk and Bat-Mite, as well as genies, such as Thunderbolt. The exact history of the 5th Dimension is hard to know, largely because of the chaotic and bizarre nature of it's reality. It is hinted that while the dimensions 1-4 are height, width, depth, and time, the fifth dimension might be.
A five-dimensional space is a space with five dimensions. In mathematics, a sequence of Nnumbers can represent a location in an N-dimensionalspace. If interpreted physically, that is one more than the usual three spatial dimensions and the fourth dimension of time used in relativistic physics.[1] Whether or not the universe is five-dimensional is a topic of debate.[citation needed]
Physics[edit]
Much of the early work on five-dimensional space was in an attempt to develop a theory that unifies the four fundamental interactions in nature: strong and weak nuclear forces, gravity and electromagnetism. German mathematician Theodor Kaluza and Swedish physicist Oskar Klein independently developed the Kaluza–Klein theory in 1921, which used the fifth dimension to unify gravity with electromagnetic force. Although their approaches were later found to be at least partially inaccurate, the concept provided a basis for further research over the past century.[1]
To explain why this dimension would not be directly observable, Klein suggested that the fifth dimension would be rolled up into a tiny, compact loop on the order of 10-33 centimeters.[1] Under his reasoning, he envisioned light as a disturbance caused by rippling in the higher dimension just beyond human perception, similar to how fish in a pond can only see shadows of ripples across the surface of the water caused by raindrops.[2] While not detectable, it would indirectly imply a connection between seemingly unrelated forces. The Kaluza–Klein theory experienced a revival in the 1970s due to the emergence of superstring theory and supergravity: the concept that reality is composed of vibrating strands of energy, a postulate only mathematically viable in ten dimensions or more. Superstring theory then evolved into a more generalized approach known as M-theory. M-theory suggested a potentially observable extra dimension in addition to the ten essential dimensions which would allow for the existence of superstrings. The other 10 dimensions are compacted, or 'rolled up', to a size below the subatomic level.[1][2] The Kaluza–Klein theory today is seen as essentially a gauge theory, with the gauge being the circle group.[citation needed]
The fifth dimension is difficult to directly observe, though the Large Hadron Collider provides an opportunity to record indirect evidence of its existence.[1] Physicists theorize that collisions of subatomic particles in turn produce new particles as a result of the collision, including a graviton that escapes from the fourth dimension, or brane, leaking off into a five-dimensional bulk.[3] M-theory would explain the weakness of gravity relative to the other fundamental forces of nature, as can be seen, for example, when using a magnet to lift a pin off a table — the magnet is able to overcome the gravitational pull of the entire earth with ease.[1]
Mathematical approaches were developed in the early 20th century that viewed the fifth dimension as a theoretical construct. These theories make reference to Hilbert space, a concept that postulates an infinite number of mathematical dimensions to allow for a limitless number of quantum states. Einstein, Bergmann and Bargmann later tried to extend the four-dimensional spacetime of general relativity into an extra physical dimension to incorporate electromagnetism, though they were unsuccessful.[1] In their 1938 paper, Einstein and Bergmann were among the first to introduce the modern viewpoint that a four-dimensional theory, which coincides with Einstein-Maxwell theory at long distances, is derived from a five-dimensional theory with complete symmetry in all five dimensions. They suggested that electromagnetism resulted from a gravitational field that is “polarized” in the fifth dimension.[4]
The main novelty of Einstein and Bergmann was to seriously consider the fifth dimension as a physical entity, rather than an excuse to combine the metric tensor and electromagnetic potential. But they then reneged, modifying the theory to break its five-dimensional symmetry. Their reasoning, as suggested by Edward Witten, was that the more symmetric version of the theory predicted the existence of a new long range field, one that was both massless and scalar, which would have required a fundamental modification to Einstein's theory of general relativity.[5]Minkowski space and Maxwell's equations in vacuum can be embedded in a five-dimensional Riemann curvature tensor.[citation needed]
In 1993, the physicist Gerard 't Hooft put forward the holographic principle, which explains that the information about an extra dimension is visible as a curvature in a spacetime with one fewer dimension. For example, holograms are three-dimensional pictures placed on a two-dimensional surface, which gives the image a curvature when the observer moves. Similarly, in general relativity, the fourth dimension is manifested in observable three dimensions as the curvature path of a moving infinitesimal (test) particle. 'T Hooft has speculated that the fifth dimension is really the spacetime fabric.[citation needed]
Five-dimensional geometry[edit]
According to Klein's definition, 'a geometry is the study of the invariant properties of a spacetime, under transformations within itself.' Therefore, the geometry of the 5th dimension studies the invariant properties of such space-time, as we move within it, expressed in formal equations.[6]
Polytopes[edit]
In five or more dimensions, only three regular polytopes exist. In five dimensions, they are:
- The 5-simplex of the simplex family, {3,3,3,3}, with 6 vertices, 15 edges, 20 faces (each an equilateral triangle), 15 cells (each a regular tetrahedron), and 6 hypercells (each a 5-cell).
- The 5-cube of the hypercube family, {4,3,3,3}, with 32 vertices, 80 edges, 80 faces (each a square), 40 cells (each a cube), and 10 hypercells (each a tesseract).
- The 5-orthoplex of the cross polytope family, {3,3,3,4}, with 10 vertices, 40 edges, 80 faces (each a triangle), 80 cells (each a tetrahedron), and 32 hypercells (each a 5-cell).
An important uniform 5-polytope is the 5-demicube, h{4,3,3,3} has half the vertices of the 5-cube (16), bounded by alternating 5-cell and 16-cell hypercells. The expanded or stericated 5-simplex is the vertex figure of the A5 lattice, . It and has a doubled symmetry from its symmetric Coxeter diagram. The kissing number of the lattice, 30, is represented in its vertices.[7] The rectified 5-orthoplex is the vertex figure of the D5 lattice, . Its 40 vertices represent the kissing number of the lattice and the highest for dimension 5.[8]
Invasion Of The Squares From The Fifth Dimension Mac Os 7
A5 | Aut(A5) | B5 | D5 | ||
---|---|---|---|---|---|
5-simplex {3,3,3,3} | Stericated 5-simplex | 5-cube {4,3,3,3} | 5-orthoplex {3,3,3,4} | Rectified 5-orthoplex r{3,3,3,4} | 5-demicube h{4,3,3,3} |
Hypersphere[edit]
A hypersphere in 5-space (also called a 4-sphere due to its surface being 4-dimensional) consists of the set of all points in 5-space at a fixed distance r from a central point P. The hypervolume enclosed by this hypersurface is:
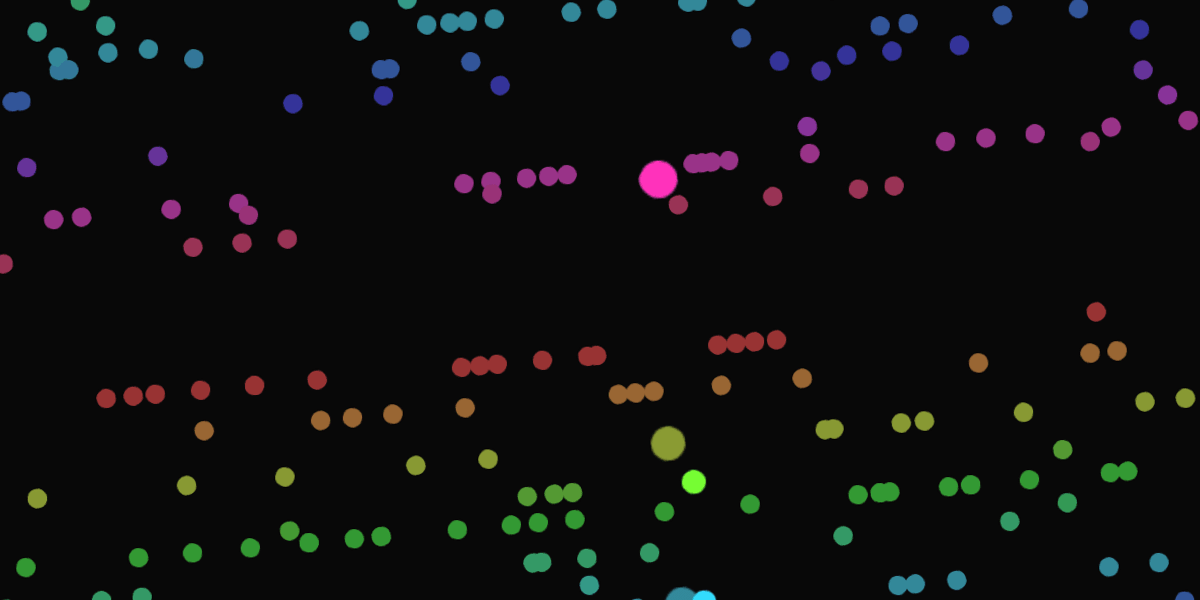
See also[edit]
References[edit]
- ^ abcdefgPaul Halpern (April 3, 2014). 'How Many Dimensions Does the Universe Really Have'. Public Broadcasting Service. Retrieved September 12, 2015.
- ^ abOulette, Jennifer (March 6, 2011). 'Black Holes on a String in the Fifth Dimension'. Discovery News. Archived from the original on November 1, 2015. Retrieved September 12, 2015.
- ^Boyle, Alan (June 6, 2006). 'Physicists probe fifth dimension'. NBC news. Retrieved September 12, 2015.
- ^Einstein, Albert; Bergmann, Peter (1938). 'On A Generalization Of Kaluza's Theory Of Electricity'. Annals of Mathematics. 39 (3): 683–701. doi:10.2307/1968642. JSTOR1968642.
- ^Witten, Edward (January 31, 2014). 'A Note On Einstein, Bergmann, and the Fifth Dimension'. arXiv:1401.8048 [physics.hist-ph].
- ^Sancho, Luis (October 4, 2011). Absolute Relativity: The 5th dimension (abridged). p. 442.
- ^http://www.math.rwth-aachen.de/~Gabriele.Nebe/LATTICES/A5.html
- ^Sphere packings, lattices, and groups, by John Horton Conway, Neil James Alexander Sloane, Eiichi Bannai[1]
Further reading[edit]
- Wesson, Paul S. (1999). Space-Time-Matter, Modern Kaluza-Klein Theory. Singapore: World Scientific. ISBN981-02-3588-7.
- Wesson, Paul S. (2006). Five-Dimensional Physics: Classical and Quantum Consequences of Kaluza-Klein Cosmology. Singapore: World Scientific. ISBN981-256-661-9.
- Weyl, Hermann, Raum, Zeit, Materie, 1918. 5 edns. to 1922 ed. with notes by Jūrgen Ehlers, 1980. trans. 4th edn. Henry Brose, 1922 Space Time Matter, Methuen, rept. 1952 Dover. ISBN0-486-60267-2.
External links[edit]
Nonlinear Estimation Toolbox 2.0 released!
This is a major toolbox release for cleaning up its API (more consistent method naming and removal of rather unnecessaryfunctionalities) to allow for a better API understanding and better implementation of new features. This release alsoincludes new estimators.
Updated Getting Started to reflect all API changes.
Also improved documentation for Estimators and Probability Distributions.
Filters now only handle the case of a single measurement. For example, Kalman filters now only accept a measurement vector, not a matrix of multiple measurements. However, processing multiple measurements can still be done by stacking measurements to a vector.
Measurement models can now only generate a single measurement with the simulate() method.
Likelihood-based filters now accept any type of measurement data, not only real-valued measurement vectors as it is the case for Kalman filters. That is, you can pass, for example, a cell array of data that is required by the likelihood function.
Various class methods are now sealed to prevent unintended overwriting.
Added new subfolders for a better structuring toolbox files. You may have to update your MATLAB PATH accordingly.
Renamed getPointEstimate() to getStateMeanAndCov().
In addition to getPointEstimate(), getStateMeanAndCov() now also returns the square root of the system state covariance matrix (the lower Cholesky decomposition) analogous to the getMeanAndCov() methods of probability distributions.
Added setStateMeanAndCov() method for fastly setting the system state without creating a temporary Gaussian distribution, e.g., in order to assign the Gaussian state estimate of a filter to another one. Note that this method does not perform input validation like setState()!
Users can now specify custom state estimate post processing via the {set,get}PredictionPostProcessing() and {set,get}setUpdatePostProcessing() methods, e.g., to implement constrained state estimation. The set post processing methods are then executed after each state prediction and measurement update, respectively.
Added several recursive update filter (RUF) variants
ERUF: extended recursive update filter (the originally proposed RUF).
CRUF: the fifth-degree cubature recursive update filter.
GHRUF: the Gauss-Hermite recursive update filter.
RURUF: the randomized unscented recursive update filter.
S2RUF: the smart sampling recursive update filter.
URUF: the unscented recursive update filter.
Dropped the analytic moment computation models used by Kalman filters and moved the analytic moment computation for the linear cases directly into LinearMeasurementModel and LinearSystemModel by introducing the getAnalyticMoments() methods.
Users can now specify a custom convergence check via the {set,get}ConvergenceCheck() methods, e.g., based on the Kullback-Leibler divergence (KLD) using the new Utils.getGaussianKLD() method.
Added getNumIterations().
Renamed setMeasValidationThreshold() to setMeasGatingThreshold().
Renamed getMeasValidationThreshold() to getMeasGatingThreshold().
Removed getLastUpdateData().
Removed the now obsolete AnalyticKF filter.
The implementation of a sample-based Kalman filter now handles the special case of equally weighted samples efficiently. That is, there is no need for a specialized implementation, e.g., for the S2KF or the UKF with equally weighted samples. This also holds for the new sample-based recursive update filters.
Renamed setNumIterations() to setNumSamplesFactors.
Renamed getNumIterations() to getNumSamplesFactors.
The used LCD-based Gaussian sampling technique can now be configured completely independently for state prediction and measurement update.
Added getNumSamplesConfigPrediction().
Added getNumSamplesConfigUpdate().
Renamed setNumSamplesByFactor() to setNumSamplesByFactors().
Removed online computation mode including the setOnlineMode() method.
Removed asymmetric LCD-based sampling mode including the setSymmetricMode() method.
Renamed setSampleScaling() to setSampleScalings().
Renamed getSampleScaling() to getSampleScalings().
Changed default name of SIRPF from 'SIR-PF' to 'SIRPF'.
Changed default name of ASIRPF from 'Auxiliary SIR-PF' to 'ASIRPF'.
Merged the PF interface into the SIRPF.
The used LCD-based Gaussian sampling technique can now be configured completely independently for state prediction and measurement update.
Added getNumSamplesConfigPrediction().
Added getNumSamplesConfigUpdate().
Renamed setNumSamplesByFactor() to setNumSamplesByFactors().
Renamed getLastUpdateData() to getNumProgSteps().
All probability distributions now have a set() method. That is, a distribution can be modified after its creation.
All default constructors now return an uninitialized distribution.
Renamed getDimension() to getDim().
Renamed getMeanAndCovariance() to getMeanAndCov().
Removed the JointDistribution.
Added check for invalid covariance matrix when trying to compute its square root in getMeanAndCov().
The getStdNormalSamples() and getSamples() methods of all Gaussian sampling techniques now return a single scalar weight in case of equally weighted samples in order to efficiently handle the computation of sample-based moments, e.g., means or covariance matrices in Kalman filtering.
Now uses a default number of 1,000 particles instead of only 100.
Added getSymmetricMode().
Added getNumSamplesConfig().
Removed online computation mode including the setOnlineMode() method.
Invasion Of The Squares From The Fifth Dimension Mac Os Catalina
Renamed getPointEstimates() to getStatesMeanAndCov().
In addition to getPointEstimates(), getStatesMeanAndCov() now also returns the square roots of the system state covariance matrices (the lower Cholesky decomposition) analogous to the Filter's getStateMeanAndCov() method.
Added setStatesMeanAndCov() method for fastly setting the system state of all filters without creating a temporary Gaussian distribution, e.g., in order to assign the Gaussian state estimate of a filter to another one. Note that this method does not perform input validation like setStates()!
Utils.getMeanAndCov() now handles the case of a single scalar weight in case of equally weighted samples (in order to smoothly work with the changed weights of the Gaussian sampling techniques).
Utils.kalmanUpdate() now additionally returns the squared Mahalanobis distance of the measurement vector.
Added Utils.getGMMeanAndCov() for computing mean and covariance matrix of a Gaussian mixture distribution.
Added Utils.getGaussianKLD() for computing the Gaussian Kullback-Leibler divergence (KLD).
Added Utils.getGaussianL2Distance() for computing the Gaussian L2 distance.
Removed Utils.baseBlockDiag().
Removed Utils.getMeanCovAndCrossCov().
Removed Utils.getStateSamples().
Removed Utils.getStateNoiseSamples().
Updated Eigen linear algebra library to version 3.3.4.
Added LinearGaussianFilter.
Added FirstOrderTaylorLinearGaussianFilter.
Added SecondOrderTaylorLinearGaussianFilter.
Added SampleBasedLinearGaussianFilter.
Added CubatureLinearGaussianFilter.
Added GaussHermiteLinearGaussianFilter.
Added RandomizedUnscentedLinearGaussianFilter.
Added SmartSamplingLinearGaussianFilter.
Added UnscentedLinearGaussianFilter.
Added IterativeKalmanFilter.
Added RecursiveUpdateFilter.
Added SampleBasedIterativeKalmanFilter.
Added SampleBasedRecursiveUpdateFilter.
Renamed BasePF to ParticleFilter.
Removed AnalyticMeasurementModel.
Removed AnalyticSystemModel.
Removed FOTaylorBasedJointlyGaussianPrediction.
Removed SOTaylorBasedJointlyGaussianPrediction.
Removed SampleBasedJointlyGaussianPrediction.
Removed KF.
Removed LRKF.
Invasion Of The Squares From The Fifth Dimension Mac Os X
Updated all 'getting started' examples to the new API, changed the used nonlinear system model, and introduced more detailed evaluation plots in the 'complete estimation example'.
Added examples for all probability distributions.
Added examples for all measurement models.
Added examples for all system models.
Added unit tests for all Gaussian sampling techniques.
Added unit tests for nearly all existing filters.
Added unit tests for all new recursive update filter variants.
Added unit tests for all probability distributions.
The general structure for filter unit tests was overhauled.